Session Aims & Scope
Digital twin (DT) has been a prevailing topic in both academics and industry, owing to the rapid development of artificial intelligence, cyber-physical systems, and digital technologies. The core idea of the DT is to develop a virtual replica corresponding to the physical entity. Through the real-time interaction between the virtual and real spaces, the system can display and analyze the state of the physical entity, and make further decisions on it. As an enabling technology of intelligent manufacturing, the DT has been used by many scholars to build the manufacturing system, that is, the DT-driven manufacturing system (DTMS). Previous research has demonstrated that DT has great potential in manufacturing. Therefore, in order to make the latest research present at the DigiTwin 2023, the conference is extending a call for papers, and a special session on Digital Twin-driven Manufacturing System is focused.
The session will focus on the following points
- Digital twin-driven process planning stage
- Digital twin-driven decision-making in the manufacturing process
- Digital twin-driven self-organization between multiple manufacturing systems
- Digital twin-driven self-adaption for dynamically changing requirements
- Digital twin-driven collaboration among multiple manufacturing systems
- Digital twin-driven autonomous interaction during the manufacturing process
- etc.
Session Chair(s)
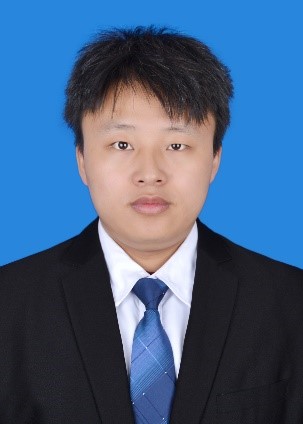
Chair
Shimin Liu
Postdoctoral fellow ,Hong Kong Polytechnic University
shimin.liu@polyu.edu.hk
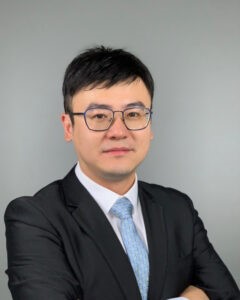
Co-Chair
Zheng Pai
Associate Professor , Hong Kong Polytechnic University
pai.zheng@polyu.edu.hk
Session Presentation
1.

Liqiao Xia
Case Western Reserve University (USA)
Title: Digital twin-driven Human-Robot Collaborative Disassembly Sequence Planning with Large Language Models in Remanufacturing
Abstract
Human-robot collaborative disassembly (HRCD) is a promising approach in remanufacturing, leveraging robot’s efficiency and human’s adaptability for disassembling end-of-life (EoL) products. However, HRCD often encounters numerous choices with uncertain outcomes, posing significant challenges. To address this issue, an HRCD sequence planning digital twin model is introduced, providing a quantitative analysis of various decisions with explanations. Initially, HRCD constraint graph is constructed for targeted EoL product based on semantic documents. Subsequently, a Dirichlet Bayesian Network (DiBN) is employed to generate feasible sequences based on the HRCD constraint graph, effectively quantifying uncertainty. Then, a fine-tuned large language model with tailored prompts is utilized to quantitatively analyze the DiBN-based sequences. The DiBN is updated with high-performing sequences from LLM, mitigating the limited knowledge about specific EoL products. Furthermore, a Generative Adversarial Network is proposed to integrate the aforementioned modules for effective training. The effectiveness of the proposed digital twin model is demonstrated through two HRCD case studies.
2.

Zhicheng Xu
The Hong Kong Polytechnic University (China)
Title: Smart Manufacturing for Ultra-Precision Machining (UPM) Technology
Abstract
In the wave of Industry 4.0 and smart manufacturing, ultra-precision machining technology is rapidly evolving to achieve higher quality, machinig efficiency, and energy savings. This course will introduce the application of the Internet of Things (IoT) and deep learning in ultra-precision machining by developing a digital twin system for real-time monitoring and analyzing energy comsumption characteristic of the machining process. With the digital twin technology, virtual models and simulations of the machining process will be explored to optimize process parameters for reducing the energy comsumprtion and achieve dynamic error compensation for higer product accuracy. In the end, the prospective of future ultra-precision machining system will be discussed.
3.

Jianan Wei
Professor
Guizhou University (China)
Title: Explainable Modeling for Equipment Fault Diagnosis Driven by Non-Ideal Operation and Maintenance Data in the Context of Digital Twin
Abstract
The digital twin process for intelligent maintenance of high-end equipment is progressing rapidly. However, due to the complexity of data collection environments and harsh operating conditions, real-time health data exhibit typical characteristics of small sample, heterogeneous, and imbalanced data. This poses significant challenges for explainable fault modeling in high-end equipment Prognostics and Health Management (PHM). This report uses explainable fault modeling of components such as bearings, tools, gears, and rotors within the digital twin framework as examples. It focuses on the treatment ideas, theories, technologies, and applications of several types of heterogeneous and imbalanced small sample maintenance data, and also explores future research directions. The findings play a crucial role in revealing the evolutionary patterns of explainable fault diagnosis modeling with small samples and provide theoretical and technical support for related fields.
4.

Qingwei Nie
Doctor
Yangzhou University (China)
Title: A multi-agent and cloud-edge orchestration framework of digital twin for distributed production control
Abstract
The demands for mass individualization and networked collaborative manufacturing are increasing, bringing significant challenges to effectively organizing idle distributed manufacturing resources. To improve production efficiency and applicability in the distributed manufacturing environment, this paper proposes a multi-agent and cloud-edge orchestration framework of digital twin for production control. A multi-agent system is established both at the cloud and the edge to achieve the operation mechanism of cloud-edge orchestration. By leveraging Digital Twin technology and Industrial Internet of Things, real-time status data of the distributed manufacturing resources are collected and processed to perform the decision-making and manufacturing execution by the corresponding agent with permission. Based on the generated data of distributed shopfloors and factories, the cloud production line model is established to support the optimal configuration of the distributed idle manufacturing resources by applying a systematic evaluation method and digital twin technology, which reflects the actual manufacturing scenario of the whole production process. In addition, a rescheduling decision prediction model for distributed control adjustment on the cloud is developed, which is driven by Convolutional Neural Network combined with Bi-directional Long Short-Term Memory and attention mechanism. A self-adaptive strategy that makes the real-time exceptions results available on the cloud production line for holistic rescheduling decisions is brought to make the distributed manufacturing resources intelligent enough to address the influences of different degrees of exceptions at the edge. The applicability and efficiency of the proposed framework are verified through a design case.
5.

Xiaojian Wen
Donghua University (China)
Title: MetaTwin: Enhancing Digital Twin with Fine-Grained Decomposition and Cross-Scale Assembly
Abstract
Digital twin technology has emerged as a powerful paradigm in the field of manufacturing, enabling virtual representations of physical assets and processes. However, the current state-of-the-art digital twin systems often face challenges in effectively capturing and representing complex systems with diverse scales and levels of granularity. To address these limitations, this paper proposes MetaTwin, a novel approach that enhances digital twin capabilities through fine-grained decomposition and cross-scale assembly. MetaTwin introduces a hierarchical structure that allows for the decomposition of a digital twin into smaller, more manageable components, each representing a specific aspect or subsystem of the physical system. This fine-grained decomposition enables greater flexibility in modeling and simulation, allowing for the representation of complex systems at different levels of detail. Additionally, MetaTwin incorporates a cross-scale assembly mechanism that facilitates the seamless integration of these decomposed components, enabling the creation of digital twins that span multiple scales and levels of granularity.
6.

Yaqian Zhang
Institute of Smart Manufacturing Systems, Chang’an University (China)
Title: Digital Twin-Enabled Task Planning and Allocation Approach for Human-Robot Collaborative Assembly
Abstract
For human-robot collaborative assembly, current research often prioritizes efficiency while neglecting the management of operator fatigue risks. To address this issue, this study proposes a digital twin framework and operational system specifically designed for human-robot collaborative assembly. Furthermore, with the optimization objectives of minimizing cycle time and reducing operator fatigue, a digital twin-enabled task planning and allocation method for human-robot collaborative assembly is proposed.
7.

Chengxi Li
The Hong Kong Polytechnic University (China)
Title: Exploring Mixed Reality’s Role in Deep Reinforcement Learning for Safe Human-Robot Motion Generation
Abstract
With the advent of Industry 5.0, the manufacturing landscape has shifted towards a human-centric paradigm, necessitating interactive assistance from manufacturing equipment, such as robots, to tackle dynamic and complex production tasks. Achieving symbiotic human-in-the-loop activities requires a robust foundation of safety measures. However, as manufacturing tasks become increasingly individualized, conventional rule-based approaches to ensure safety fall short due to their inflexibility and lack of synergy. To address this gap, our study presents a novel mutual-cognitive safe interaction approach by leveraging Mixed-Reality devices and Reinforcement Learning. This approach integrates key elements including worker visual augmentation, robot velocity control, Digital Twin-enabled motion preview, collision detection, and Deep Reinforcement Learning-based robot collision avoidance motion planning, all facilitated through Mixed Reality technology. By combining these components, the proposed approach aims to enhance safety while enabling effective interaction and collaboration.
8.

Yicheng Sun
Donghua University (China)
Title: Research on Digital Twin Temporal Intelligent Agent Based on Large Language Model
Abstract
Digital Twin (DT), as an efficient technology for virtual-physical interaction, has demonstrated significant application potential in various industries. Intelligent agent-driven digital twin systems excel in analysis, decision-making, and control, making them highly suitable for manufacturing resource scheduling, diagnostic decision-making, and other requirements. However, current intelligent agents have notable deficiencies in adaptability, data utilization, and interpretability. This limitation undermines decision security and acceptability, creating barriers for user intervention. Therefore, we introduce a DT multi agent architecture driven by Large Language Models (LLMs). Agents perceive the characteristics of physical systems, particularly their temporal characteristics, by integrating data from various modalities. Multiple agents achieve insights through specific interaction mechanisms, while maintaining traceability. To showcase the advantages and characteristics of this architecture, we developed a typical application scenario for equipment maintenance.
9.

Xinyu Liu
School of Engineering, Ocean University of China (China)
Title: An Adaptive Dynamic Digital Twin Approach for Product Performance Optimization
Abstract
Digital twin has been gradually explored and applied in the field of product intelligent optimization. As a technology that maps physical entities to virtual domains, digital twin optimization models can be constructed to update and solve the model in response to the change in operation state. However, the correlation between the updated model and the original model is not considered in the above process, and the updated model needs to be re-solved. This leads to the poor adaptability of the current method. Therefore, this paper proposes an adaptive dynamic digital twin approach for product performance optimization. By constructing the DT optimization model based on the Gradient Boosting Regression Trees (GBRT), which can infer and update the mapping relationships between the parameters and indicators to be optimized. Subsequently, a dynamic optimization strategy based on Adaptive Non-dominated Sorting Genetic Algorithm (Ad-NSGA) is proposed. Based on the evaluation of the degree of model correlation, it realizes the dynamic update of the population and adaptive search for optimization. Finally, the effectiveness of this approach is verified through a case study on the bogie system, which has better adaptability in dynamic optimization.
10.

Haozhi Xiong
Postgraduate student, Wuhan University of Science and Technology (China)
Title: Digital twins- based Multi-Robot Machining Method for Cabin-Like Components
Abstract
As an important component of aerospace equipment such as missiles, rockets and satellites, there are many complex intersecting characteristics and narrow feasible space inside the cabin components, which bring great challenges to the processing of its inner wall. The processing efficiency and intelligence level of manufacturing equipment are difficult to meet the rapid and high-quality development needs of aerospace equipment. To improve the machining efficiency and intelligent level of cabin component manufacturing equipment, a multi-robot intelligent collaborative machining method based on digital twin was proposed. The digital twin framework of virtual space, physical space and information data layer of robot collaborative processing system with different functions is constructed. The design of twin data model such as twin data source and type, data fusion and data storage is elaborated in detail. The optimal control and decision-making model of multi-robot collaborative processing is established. The software and hardware platform of multi-robot intelligent processing is developed. The virtual-real mapping and interaction are realized, and the on-line monitoring of processing system is realized. Taking the precision milling and measurement process of a certain type of aircraft cabin as a case for verification and analysis, the processing route is optimized, the processing efficiency is improved, and the feasibility and efficiency of the method are verified.
11.

Yanfeng Kuang
Postgraduate student, Wuhan University of Science and Technology (China)
Title: TransK-CNN: Convolution based on kernel fused transformer for artery vein classification in fundus images
Abstract
The early screening of the retina can effectively prevent certain diseases, and the segmentation of blood vessels in fundus images can enhance the efficiency of screening. However, further segmenting these blood vessels into arteries and veins poses a more complex challenge. While numerous scholars have proposed some deep learning methods for fundus vessel segmentation, most of these approaches primarily focus on convolutional methods which are insensitive to pixel relationships. To address this limitation, this paper proposes a unique approach that combines both convolutional kernels and transformers. Unlike other methods that combine these two techniques, our attention mechanism is calculated within the convolutional kernel framework to overcome the computational limitations associated with transformers in image analysis tasks. The proposed method is validated on the RITE and HRF datasets. Moreover, the RITE dataset achieved a sensitivity of 0.9698, specificity of 0.9491, accuracy of 0.9604, and F1 index of 0.9647. Similarly, the HRF dataset yielded impressive scores with a sensitivity of 0.9797, specificity of 0.9726, accuracy of 0.9765, and F1 index of 0.9785.